With Augstín Da Fieno Delucchi, Principal Data & Applied Scientist at Microsoft
Below is a complete transcript of this episode
Stephanie Harris 0:16
I’m Stephanie Harris and I will be your host today for this episode of global ambitions. My guest today is Agustín Da Fieno Delucchi who is the Principal Data and Applied Scientist at Microsoft. Agustín, welcome to the program.
Agustín Da Fieno Delucchi 0:30
Thank you for having me here. It’s a pleasure to be here.
Stephanie Harris 0:34
We’re very excited about our topic today which is using AI in the internationalization of software. So to get started, can you give us a brief background of yourself?
Agustín Da Fieno Delucchi 0:45
Well, I’ve been in the localization industry for about 22 years now. I started as a terminologist and doing some linguistic control for Windows 98 back in those days. And since then I’ve been ago have gone through many different roles in the localization industry localizer or translator. And then I moved more into the engineering aspects of it. My background is in computer science, but always my work is being related to internationalization because when I found this world, I just fell in love with it, and it became one of my passions. So, since then I have many different roles. In all these roles I’ve always been involved in database development in finding data-related solutions to the problems we have. Recently, a year and a half now, I started this position as a data scientist specialized in the area of internationalization.
Stephanie Harris 1:46
All right, well, I guess let’s go ahead and just jump right into it then. With what you’re working on now, what do you find is the most interesting and challenging thing?
Agustín Da Fieno Delucchi 1:56
Like many other areas, work, or industries the digital transformation and the use of AI starts to come up and it’s becoming really the hot topic. And we are seeing many companies investing in it and trying to apply this. It is exciting what can be done. And there are many scenarios that we can start seeing helping validation of code, or validation of translation.
Agustín Da Fieno Delucchi 2:23
We know that in the aspects of the industry, the most advanced aspect of this is machine translation. I mean, I recall 20 years ago I started doing some trials with translating so it’s certainly mature now for our space but is it the most common one?
Agustín Da Fieno Delucchi 2:41
I think they now, and this is what I’m trying to focus on, is how do we embed it into the business aspects of the world? So that’s what I’m focusing on. How do we gain efficiencies through the entire internationalization pipeline from the creation of code to when the products are translated and go to the customers and close in the circle? So that’s what I’m focusing on, and involves things like, yes, validating code, validating translations, but I think that one of the key aspects in the industry is helping translators with context. It is a big thing, we know that context is everything in our world. So I think that’s something that certainly requires attention.
Stephanie Harris 3:29
Yeah, yeah, that’s huge. As you’re embarking on this work, what’s one of the biggest mistakes or challenges or misconceptions you’ve seen in getting that actually done? In getting the context available and getting those inputs for your translators, as they go through the process.
Agustín Da Fieno Delucchi 3:48
Yes, absolutely, like everything else, particularly the new things, there are many challenges and other constraints. I think one of the main challenges is to have the right amount of data that is consistently recorded across all the layers of the business. So we know that in the case of translations, yes, we have a lot of data, and we can certainly use that. But when we talk about metadata related to the transactions that are part of the process, as a translator changes the string, as the source string changes, as they are word counting, all the finances involved, all these different things done in the engineering side. So all these are records that should represent actions.
Agustín Da Fieno Delucchi 4:39
So, what, what tends to happen is that this is happening, is not just a problem in the localization industry. Is that different layers of this ecosystem track data, or different levels, so try to unify that and try to get a consistent, you know, data set for all your needs becomes a big challenge, Because it requires, in some cases, dramatic changes into how you store, and your solutions involving how to cater for the level of granularity in the data.
Agustín Da Fieno Delucchi 5:16
Now, particularly for models that involve AI and machine learning, as one of the applications, you require big amounts of data, but good data. So the data has to be aligned and properly recorded to satisfy certain needs and should have the right annotations. In some cases, you need to go back and invest in labeling certain records to be able to develop this model. So I think that’s where the big challenge is. To modify these business layers in a way that you can have consistent data traveling all along the pipeline. And by the way, there is no industry standard for that.
Agustín Da Fieno Delucchi 6:01
Right, so for translation we are more advanced because there is an industry-standard, you know, we have a really good standard to have, but when we talk about transactional data, how to record it… So it’s hard, and that’s one of the sometimes key challenges that we have. Even within a client and a service provider. So, that’s, that’s what I would see as the main challenge.
Stephanie Harris 6:26
Yeah, so when you’ve been working with clients and service providers, what’s one thing that you’ve seen that’s actually worked really well and that you would recommend people try?
Agustín Da Fieno Delucchi 6:38
Well, I think first of all is important to align priorities. Understand what are these common problems that we see on each side and which of those are going to give us the best results for the business. So it’s important to prioritize because you can do many things with AI. But if they don’t actually give you business results… Then, well you can have fun in your spare time. But I think it is important to invest properly in evaluating the main problems of the business.
Agustín Da Fieno Delucchi 7:08
So, without prioritization and alignment that you can start peeling the onion and going into, OK, let’s try to focus on these very important problems how do we achieve that. So, little by little, so you start selecting what are these components which we now require data on both sides and how does that data travel. And when you do that, then, it’s a smaller scale, it’s manageable, and not so overwhelming as looking at the entire system.
Agustín Da Fieno Delucchi 7:39
Because you, you look at that and the entire ecosystem and it’s just humongous. So you really want to peel the onion, set the right priorities, and when you do that… A particular example that we are currently working on is related to providing better context for translators in the case of entities found in a given string that do not necessarily require localization. And with what level of confidence. To tell them, I have this confidence that they should not be translated. And in some cases, you just lock it for them. So that’s one clear example of when things were well when the data travels well but also the business priorities are default.
Stephanie Harris 8:22
Okay, that’s a very good example, I you know we didn’t go over this question but I was just going to ask, is there anything that you see in the future or coming up soon that you’re excited about the possibility of being able to do? That maybe we couldn’t do in the past but now with the improvements in technology, suddenly it seems like it might be possible?
Agustín Da Fieno Delucchi 8:42
Yes, I think that one big area that is rising right now is, an emerging way of doing things, particularly in AI, is content generation. So whereas in general, machine learning particularly now is very good at summarizing and extracting information from something that exists. Terminology mining, or just finding the key terms or summary from a document. We’ve seen examples like that. It’s extreamly good at that.
Agustín Da Fieno Delucchi 9:11
The space that I think is probably going to become really important is that one of using AI to generate context that can facilitate things in the pipeline. For example, is the providing a revision of the source code that can work better for internationalization, for example. And they’re starting to appear some solutions there. Then that’s one option.
Agustín Da Fieno Delucchi 9:12
And in the same case, it goes along the way, in terms of different ways of approving, summarizing status reports here and there. All these things that can be generated and created based on that. And then also, you know, it’s like, providing also already some reviews of the… taking the work of reviewing the translation.
Agustín Da Fieno Delucchi 10:04
So I think that that area of content generation is particularly interesting, and not only for localization but yes it can be used.
Stephanie Harris 10:12
Yeah, now that’s very, very exciting. Well, I think that’s about it for today so thank you so much for joining us here.
Agustín Da Fieno Delucchi 10:20
Thank you so much, you know, it’s been a pleasure and honor to be here.
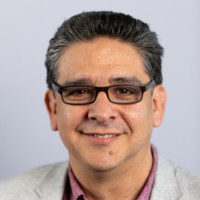
Agustín Da Fieno Delucchi
Principal Data & Applied Scientist at Microsoft