With Serge Gladkoff, CEO of Logrus Global
Below is a full transcript of this episode.
Antoine Rey 0:25
I’m Antoine, and I will be your host today for this global ambition podcast. And my guest today is Serge Gladkoff, who CEO of Logrus Global. Today our topic is going to be around NMT and what we call the last mile. Serge, welcome to the program.
Serge Gladkoff 0:43
Thank you. Thank you for inviting me here.
Antoine Rey 0:45
So, straight into our topic today, there’s suddenly a lot of hype in the industry and everybody seems to be using NMT these days. However, the closer we get to the goal of perfect translation through NMT, the harder it seems to be getting to that last mile, and there seem to be a number of misconceptions. Can you talk to us a little bit about that?
Serge Gladkoff 1:08
This is the most interesting topic of our days. It’s really a wonderful time when we have something new. Because for many many decades translation seemed to be well known and simple, but now we are at the point where a lot of new things are happening. Really, we actually have this wonderful technology that’s giving us amazing results, but we don’t have artificial intelligence at all.
Antoine Rey 1:37
Explain that a little bit for our listeners because I’m sure, people might disagree.
Serge Gladkoff 1:42
They may disagree but fortunately, the Massachusetts Institute of Technology has put it in their recent article GPT-3, “The greatest trick the AI has pulled off, is to convince the world that it exists.” This technology does not think, does not process meaning. It is a wonderful way of working with embeddings and processing a huge amount of data and corpus and producing wonderful results, which are useful. But the question is how do we use them? And what are these results actually are?
Antoine Rey 2:19
Our 17 billion parameters don’t mean artificial intelligence, right?
Serge Gladkoff 2:24
Well, let me tell you this. The GPT-3 is an example of, likely, the most advanced neural network to date. It is huge, is trained on nearly all corpus that they could find in the world. Like, I don’t even know how many terabytes of data, which is taken from everywhere from books, newspapers, web, you name it. It does have 17 billion parameters, and parameters are simply weights in the neural network, but the thing is that it’s actually a simple model which is simply trained on very large corpus of data. And it’s nice it generates this smooth text but it does not have a mind. That’s a fact.
Antoine Rey 3:05
And so you’re saying, in this case, there’s really a requirement for skilled people, and that human in-the-loop intervention during that last mile, right?
Serge Gladkoff 3:16
As we’ve worked with machine translation we, of course, admire the results, but what I would like to point out is that today, when this technology is all around us and everywhere, all of us have to be both enthusiastic, and skeptics of this technology. Because on one hand, we should admire the way it works. And it’s actually very interesting how it works and how to apply it. As soon as you’re trying to understand how it works, you see that it does not process the meaning.
Serge Gladkoff 3:47
It’s like, this is a technology that allows you to actually capture the meaning, which is in the corpus. But it’s only realized through its usage. So, there is no intelligence as such. What artificial intelligence is basically a neural network that analyzes the traces of a bunny on the snow. You see that there is an animal, which has four legs, and it jumps far, but there is no way to imagine what this bunny looks like. You can only say that this is a relatively small animal. You will never see what’s inside of that bunny. So when they… the human language leaves traces of thoughts and the artificial intelligence process these traces, but it does not reconstruct the meaning at this point. So we are very far from so-called general artificial intelligence.
Antoine Rey 4:43
And this is where you need the skilled post-editors to bring that meaning, and that final mile?
Serge Gladkoff 4:49
When we work with MT post-editing with an MT output and trying to present it. You will see immediately that the output looks nice. And the business of making words flow smoothly is now is to a very good extent, but it does not process meaning. And especially in languages that are far from English, you see a lot of problems with the meaning transfer, that is, severe errors of the meaning transfer are actually making their way to the output, naturally, because the machine does not analyze the meaning.
Serge Gladkoff 5:29
So, if you take the output of MT, which is a mindless machine, and the output is mindless as well, you need to put the mind to this result to get really good meaningful results. And “what is really good?” is of course the question. Which many people are trying to answer with premises such as something taken from the wall and good enough translation. But the truth is, and actually, this took several years for the industry to realize the truth, is that in order for a practitioner, for the client, for the practitioner to actually make good use of machine translation in its current form in neural networks, and realize all the productivity gains, and all the financial gains that we want to realize you actually have to apply these skilled language professionals to the MT output.
Serge Gladkoff 6:26
So that is one of the biggest misconceptions, is that with MT, you can get by with low cost low skilled labor, and here you and it is lengthy and painful. No, that that is actually quite the opposite. So again, in order for the client to implement the gains of machine translation, you need to work with highly skilled people. If you’re not working with a professional, trying to crowdsource those post-editors, you will end up with a situation when the mindless machine is doing the output and then mindless people are trying to edit this output not understanding anything that they’re doing.
Serge Gladkoff 7:03
And that is unfortunately the situation that we see a lot. Recently I was meeting with a client who’s saying that I understand that this is not so. But my internal customers have that vision. Well, my answer was that you need to educate your internal customers because they probably want it to be done quickly and to a large scale and without that much cost, but I don’t think that they want to get rubbish, right?
Serge Gladkoff 7:32
Let me give you an example. You have a BMW okay? You know that you have a quality car, and all you need to do is to learn how to drive. But in some countries, they’re very low-quality cars that break all the time. In order to drive that car, you actually need to be a mechanic. Because it breaks, because it breaks on the road, and he doesn’t know what to do. Okay, so with machine translation it’s the same kind of thing. Yeah, you actually need highly skilled labor to use MT to watch for the quality that you need to get in terms of the client requirements.
Serge Gladkoff 8:13
The client said, “I don’t want the final quality.” Okay, I understand that you don’t need a poem, but you probably don’t want to really get a marketing text with a lot of blunders that are completely unacceptable in terms of the, really, meaning transfer. I mean this cannot be said. To monitor the key output of the machine that’s changed, well, you can get smooth text, but you need to read 100% to find five or six sentences, which really need to be corrected. Okay? Because if you don’t correct them, people will be laughing and your reputation will suffer. And then not because it’s a huge terrible error, but because it’s a factual error. A dumb error. And the people will laugh at your company for that.
Antoine Rey 8:19
But what is the impact then of NMT over the terminology and maybe even the translation memories that are in place, and that we are using?
Serge Gladkoff 9:11
It is another huge problem. So-called post-editors are editing the text, and they are taken on assumption that they should be a low skill low-cost labor. We are ending up with all those blunders and errors making their way into the translation memory. The literal imperfections are not corrected at all. And we’re seeing that with some of our clients.
Serge Gladkoff 9:38
When the translation memory is getting polluted very quickly and deteriorating. The quality of translation memory and that really puts you into a dead-end where translation memory stops making sense. Some of our clients will say, “It will not be a benefit to put that into translation memory.” That is actually something that you cannot control. Because if you are using that quote-un-quote translation, that will make its way into translation memory, and at the end of the day you will see that you will have to check 100% of the text in the translation memory. So matching will stop working at all, and that’s not a good thing because it raises the amount of work you have to do. There are no matches anymore which you can trust. They are wrong. So you have to check 100%. That is the situation, which resulted already with some of our clients, unfortunately.
Antoine Rey 10:38
And what’s happening with the terminology side of things because if I understand well, an NMT is not designed to handle terminology.
Serge Gladkoff 10:47
The NMT by definition by design works well when there is a huge corpus of text, and that corpus is best when it’s accurate, and it’s good content and diverse and in abundance. So, if you have a lot of that, the result is good, in the sense that it’s usable and it can work with it further. But by that mechanics, the terminology, cannot be handled because terminology is actually less frequent words in the text not most frequent words in the text. That’s one thing.
Serge Gladkoff 11:24
And another thing is that terminology, just as language in general, is ambiguous. That is, it contains things that are not so straightforward. And they play different roles in different contexts and synonyms… terms have synonyms. So, I’m sure that those of you who have been working with terminology professionally, know that TPX standard has the Concept at the top, and only then they have the terms for the language. So it’s one too many relationships between the concept, and the term in one language and then there are many relationships with the terms in different languages. So, the terminology is ambiguous as well.
Serge Gladkoff 12:09
So there are ways to clean the corpus. Like some people are trying to simply delete all of the so-called incorrect terminology from the text. But the thing is that if you tweak the training corpus that way, then that engine will not be able to process any of the synonyms and it will be only using the terms in one way, which will make all debatable quality and approach is really something used different several different ways. It’s like when you put the guy in the bed and cut off his head. Then something which is left on the bed is not really a nice looking, you know, picture.
Antoine Rey 12:56
Serge thank you very much for explaining some of those misconceptions about NMT and giving our listeners some ideas on how to get around some of those with a skilled human in the loop and working even closer with terminology and translation memories as you use NMT. I’m sure there might be more questions from our listeners and I would encourage them to reach out to us and to Serge in this case. So thanks very much for joining us on this program, and we look forward to talking to you again very soon.
Serge Gladkoff 13:27
Thank you very much. It was a pleasure.
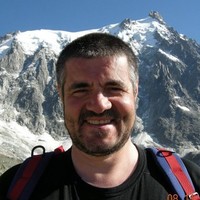
Serge Gladkoff
CEO @ Logrus Global